I am an Assistant Professor in the Computer Science Department at Worcester Polytechnic Institute (WPI) and a proud member of the Cake Lab! Broadly, I am interested in designing systems mechanisms and policies to handle trade-offs in cost, performance, and efficiency for emerging applications. Specifically, I have worked on projects related to cloud/edge resource management, big data frameworks, deep learning inference, distributed training, neural architecture search, and AR/VR. My recent work has a strong focus on improving system support for deep learning and on the practical applications of deep learning in AR/VR.
I completed my Ph.D. at the University of Massachusetts Amherst advised by Prof. Prashant Shenoy. Before that, I received my B.E. from Nanjing University and was an exchange student at National Cheng Kung University.
- Jean-Baptiste Truong (co-advised with Robert Walls) - Master, 2021. Thesis: Protecting Model Confidentiality for Machine Learning as a Service. Current employment: Deep Learning Engineer at Geopipe, Inc..
- Dr. Xin Dai (co-advised with Xiangnan Kong) - PhD, 2022. Thesis: Redesigning Deep Neural Networks for Resource-Efficient Inference. Current employment: Research Scientist at Visa Research.
- Dr. Sam Ogden - PhD, 2022. Thesis: MiseEnPlace: Fine- and Coarse-grained Approaches for Mobile-Oriented Deep Learning. Current employment: Tenure-track Assistant Professor at California State Monterey bay.
- 02/27/2023: 😁 I received the tenure decision letter! I will be promoted to Associate Professor and awarded tenure effectively July 1, 2023.
- 01/28/2023: [Paper] Two papers have been accepted to CCGrid 2023. It is great to see LayerCake, the last piece of Sam's thesis got accepted! The other paper on gradient accumulation is conditionally accepted. [Update:02/27/2023] The revision is successful and the revised submission has been accepted!
- 01/13/2023: 💰 [Grant] I received the NSF CAREER award to work on designing a specialized edge for AR.
- 12/09/2022: [Paper] Our paper on dual-camera lighting estimation for virtual try-on applications has been accepted to HotMobile 2023!
- 10/26/2022: [Talk] I gave a keynote speech at Mass Academy of Math and Science during the Conversations about STEM 2022 event, about my research on cloud-based distributed training and edge support for mobile AR and personal experience in grant writings and in STEM.
- 10/04/2022: [Paper] Our paper Funcpipe has been accepted to SIGMETRICS' 23!
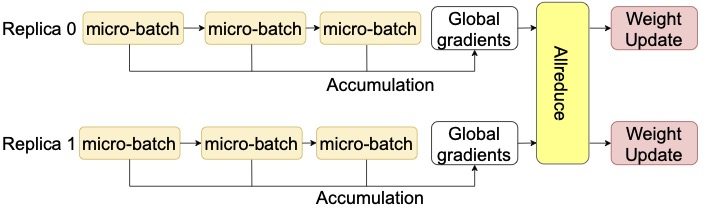
Measuring the Impact of Gradient Accumulation on Cloud-based Distributed Training
THE 23rd IEEE/ACM International Symposium On Cluster, Cloud and Internet Computing (CCGrid'23)
Though GA is a commonly adopted technique for addressing the GPU memory shortage problem in model training, its benefits to model training have not been systematically studied. This paper evaluates and summarizes the benefits of GA, especially in terms of cloud-based distributed training scenarios, where training cost is determined by both execution time and resource consumption.
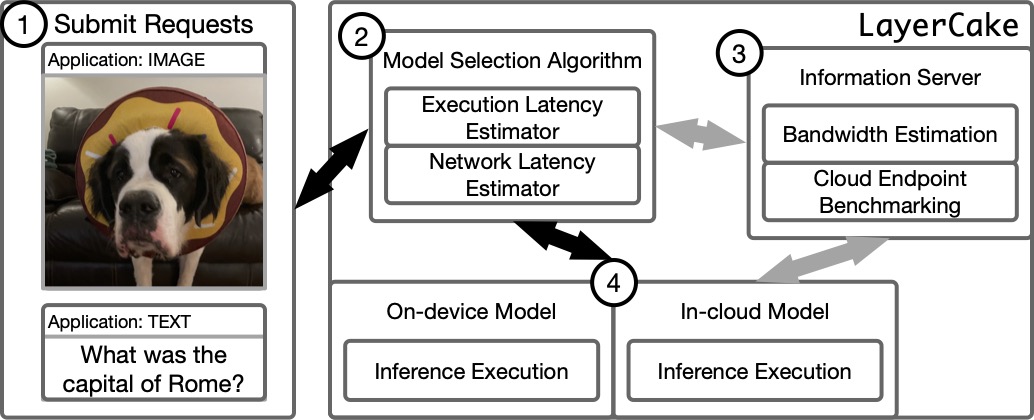
LayerCake: Efficient Inference Serving with Cloud and Mobile Resources
THE 23rd IEEE/ACM International Symposium On Cluster, Cloud and Internet Computing (CCGrid'23)
The landscape of DL inference has changed drastically since our first paper on mobile deep inference! Many mobile-oriented models have arised and more apps are leveraging DL models. This paper considers the dynamic inference execution environment and schedules the request to the best-available resource.
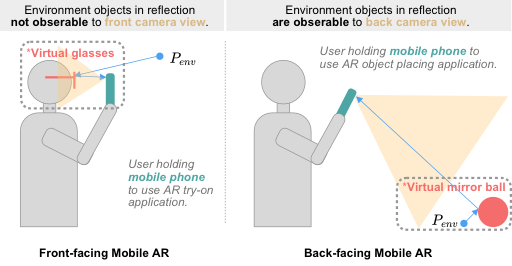
Multi-Camera Lighting Estimation for Photorealistic Front-Facing Mobile Augmented Reality
The Twenty-fourth International Workshop on Mobile Computing Systems and Applications (Hotmobile'23)
We demonstrate the promise of dual-camera lighting estimation in improving rendering effects for virtual try-on AR applications. Furthermore, we also show that an existing SToA lighting estimation model can't fully utilize the enlarged camera view.
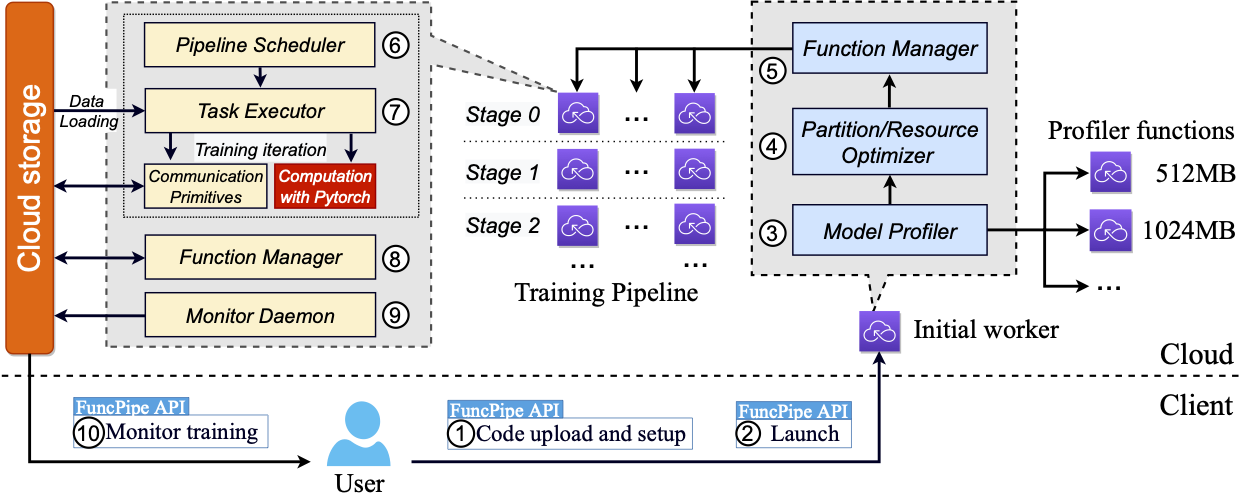
FuncPipe: A Pipelined Serverless Framework for Fast and Cost-efficient Training of Deep Learning Models
Proceedings of ACM SIGMETRICS, 2023 (SIGMETRICS'23)
FuncPipe co-optimzes model partition and serverless resource allocation to reduce memory consumption and also relieve communication burden in distributed training. Further, we designed a pipelined scatter-reduce to simultaneously utilize downlink/uplink bandwidth.
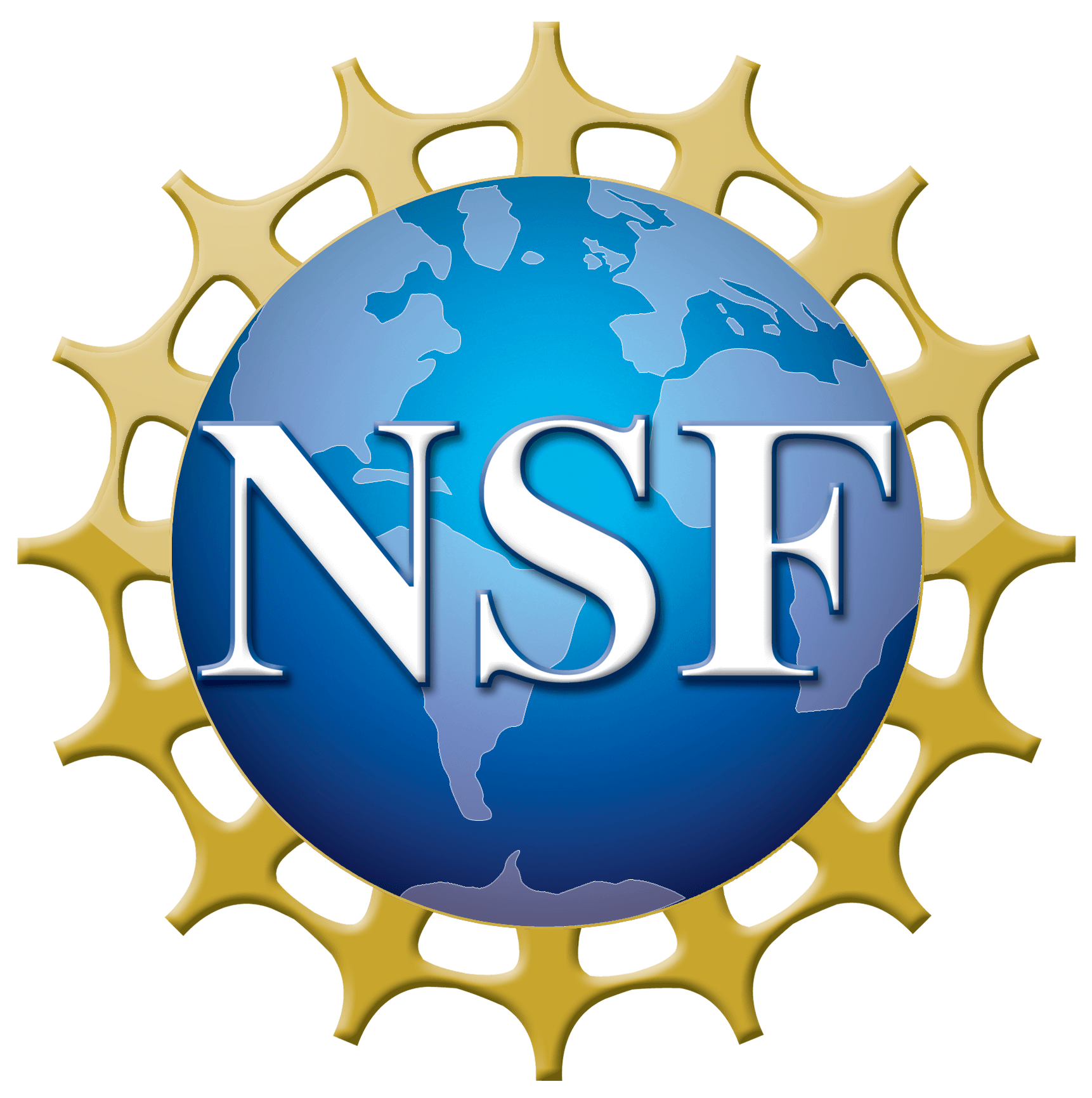
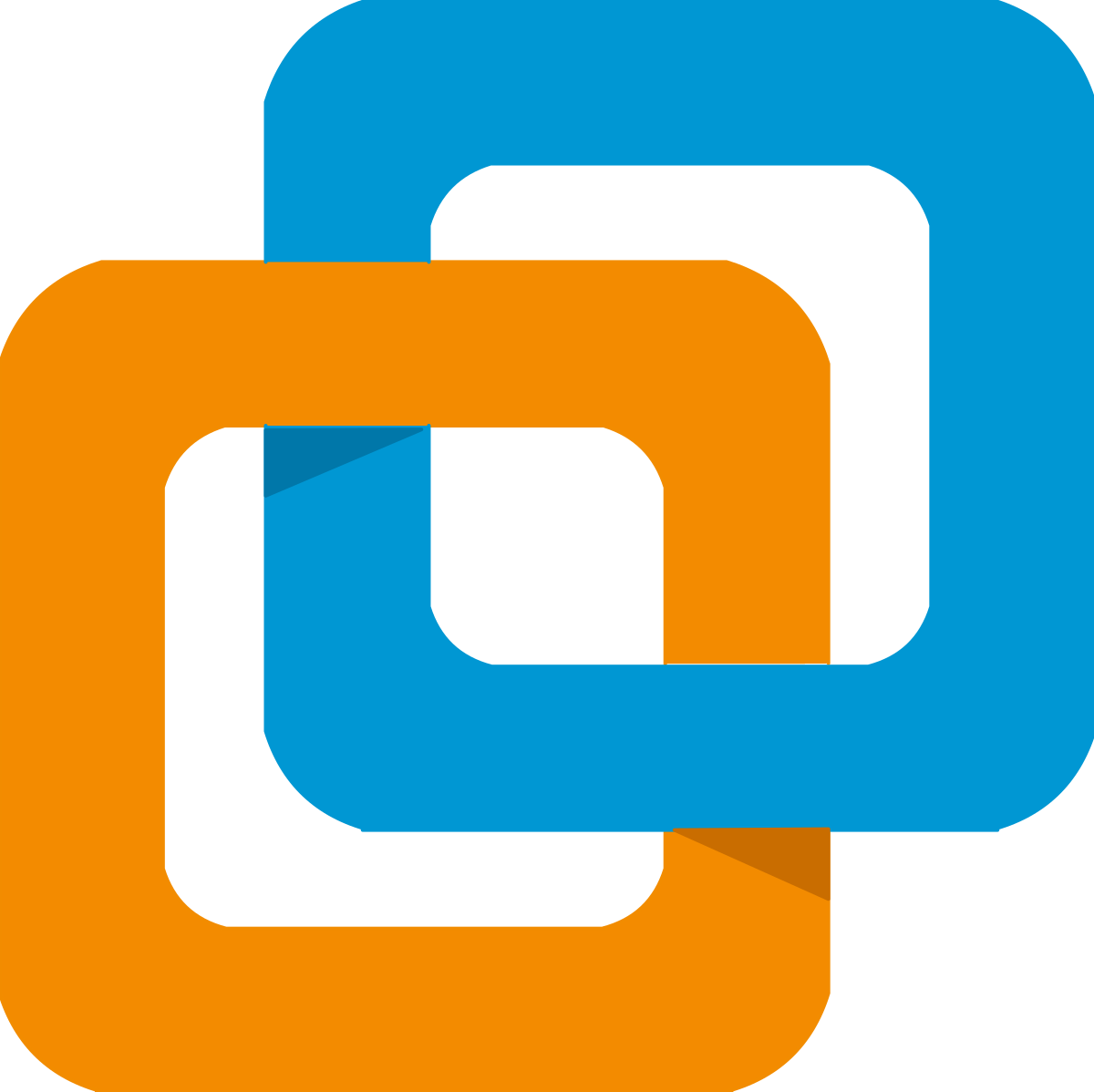